Manufacturers can sift through the GenAI hype and realize value from industrial AI by addressing these fundamental questions.
Artificial intelligence (AI) is often touted as the panacea to data challenges and the uncertainty that comes with building intelligent products and services. While most enterprise implementations have been focused on solutions in HR, financial modelling, IT operations and even digital products, these do not extend naturally or easily to physical products, assets and the built environment.
In the ISG Market Lens AI Study, we found that more than 90% of the time, manufacturers implementing AI are doing so in hopes of business growth, innovation and efficiency in that order. But manufacturers lag behind typical organizations in other industries when it comes to the number of AI-enabled applications being implemented. This lag has real implications for intelligent products, Industry 4.0 and, more recently, Industry 5.0, with the workforce being a major consideration.
The factors that are hindering AI implementations in the manufacturing industry include:
The lack of IT-OT convergence, the effective and efficient intersection of people, process and technology across the organization
Data issues, including data management, data quality and governance
The difficulty of capturing and contextualizing domain knowledge for machine learning and teaching purposes
The lack of appropriate approaches and tools for implementing AI
The skills and capabilities gap
Another challenge is the physicality of problems in such industries – defects in cars, unsafe food, etc. – as they cannot be merely “scrubbed” and “coded” in binary or digital methods. These problems can have immediate and significant consequences, including product defects, toxic waste spills and even human injury/loss of life.
This is what the power of industrial AI addresses. Industrial AI is a term coined by Dr. Jay Lee who was the founding director of the National Science Foundation (NSF) Industry/University Cooperative Research Center (I/UCRC) on Intelligent Maintenance Systems. Even with the advent of the latest technologies and digital skills in manufacturing, the gaps that exist in intelligent products and services cannot be bridged without industrial AI applications.
What Is Industrial AI?
At ISG, we define industrial AI as the use of AI technology in digital initiatives and processes in asset-heavy industries to improve efficiency, productivity, quality and decision-making. Industrial AI helps predict outcomes and automate tasks across the connected value chain from “concept to design” and all the way to aftermarket and end of life.
Industrial AI can take many forms; it can be AI that is autonomous, predictive, prescriptive or generative. Specific applications of industrial AI are being used in various industries, including manufacturing, energy, transportation, healthcare and the physical product space. For an understanding of what a use case looks like in product development, read our recent article Leveraging Industrial AI for Product Design.
Recognizing and defining the problems you are trying to solve in your journey to modern industrial manufacturing is not enough to know exactly where and how to adopt industrial AI. A reference architecture that is built around the capabilities of AI will be needed to shape the discovery, organizational structure, adoption and potential value of an industrial AI use case. An industrial AI reference architecture is the means to scale industrial AI across a manufacturing enterprise and keep initiatives from getting stuck in pilot purgatory.
Please fill out this form to continue.
Where to Begin Industrial AI Adoption
Like other technologies, AI will likely become a commodity for most organizations. The implementations of industrial AI that exist today are customized and sit within one of four key manufacturing areas: customer experience, business operations, engineering research and development or IT and DevOps. As you can see in Figure 1 below, the potential in industrial AI adoption is understanding the overlap between these areas and how to leverage potential synergies for building and scaling intelligent products, connected customer experiences and application modernization.
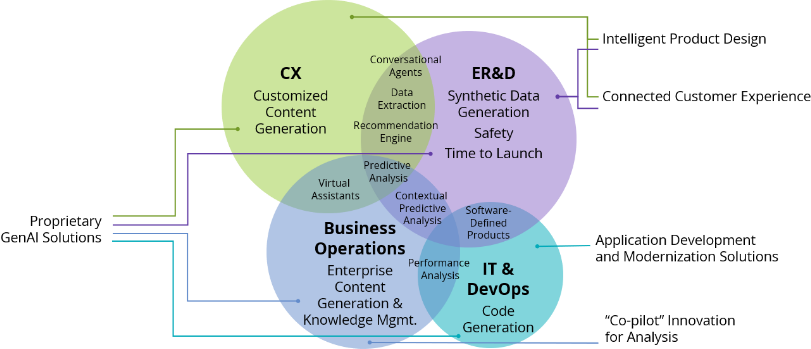
Figure 1: Where to Begin Industrial AI Adoption
As you can see, most successful use cases – including data extraction solutions, virtual assistants and predictive analysis – are cross-functional, with benefits in more than one area.
A challenge for manufacturers is figuring out which industrial AI use cases can help them create a competitive advantage. Determining the purpose of investing determines the approach to AI adoption.

Figure 2: Business Value Streams of Industrial AI
The most successful uses cases will achieve value in at least one of the following business value streams:
- Refining products: using AI to augment existing products and services to improve customer satisfaction and experience, improve productivity and decrease costs.
- Innovating new product development: building an industrial AI native product or service to find new growth areas, increase revenue and unlock new revenue streams.
- Rebuilding processes: harnessing industrial AI to re-imagine processes with native AI by design, improve time-to-value and time-to-market and create human-in-the-loop processes.
- Supporting existing processes: using AI to drive efficiencies, improve customer satisfaction and experience, create human experience-centric processes, improve productivity and decrease costs by unlocking hidden opportunities.
4 Questions to Steer Your Industrial AI Journey
Determining the purpose of investing determines the approach to AI adoption.
To understand which value stream to select and how to proceed on your industrial AI journey, ask yourself these four critical questions:
- Where can industrial AI create differentiation for your organization?
Get clear that the purpose of your organization is aligned with investing in AI and its core business proposition. By first understanding the differentiation you are aiming for, it will become clear which parts of the organization need to be developed. For example, using automated chatbots to improve customer engagement will require different investments than embedding intelligence in the development of a physical/digital product. - How will the areas of value differentiation from industrial AI impact your existing operating model?
Answering this question requires looking beyond the enterprise’s technical aptitude, capabilities and infrastructure and across its people, process and data. Manufacturers will need to recalibrate their buy-vs-build strategy to not only scale and sustain industrial AI adoption but also to accelerate the time-to-value of their investments and safeguard the differentiation as an economic moat. - How do you address the safety, regulatory and compliance requirements while adopting industrial AI and how can you sustain such a path forward?
Manufacturers already have a sense of the difficulty of putting in place governance measures that ensure high-quality data is available for business intelligence, analysis and reporting. These challenges will only grow when it comes to governing and managing industrial AI applications. The ramifications of an ineffective means of governing industrial AI outweigh any potential business value. - How can manufacturers leverage their current digital investments to fast-track time-to-value from industrial AI investments?
Manufacturers have invested in building solutions like digital twins and digital threads as part of their digital transformation agendas. Digitization, data and information exchange have been at the heart of these investments and are meant to serve as the digital foundation and data management guardrails to accelerate industrial AI adoption.
But many solutions likely operate in silos that cater to specific business problems or just a portion of the value chain. Manufacturers need to evaluate the maturity of their existing solutions and their capability to augment them with industrial AI. The results promise to be much greater across the entire value chain than their current state.
ISG helps enterprises navigate the changing manufacturing market and responsibly implement digital technologies and ecosystems to support their future product goals. The ISG Industrial AI Reference Architecture pulls it all together to help manufacturers on their industrial AI journey build modular and composable solutions. Contact us to find out how we can help.